With a Trace
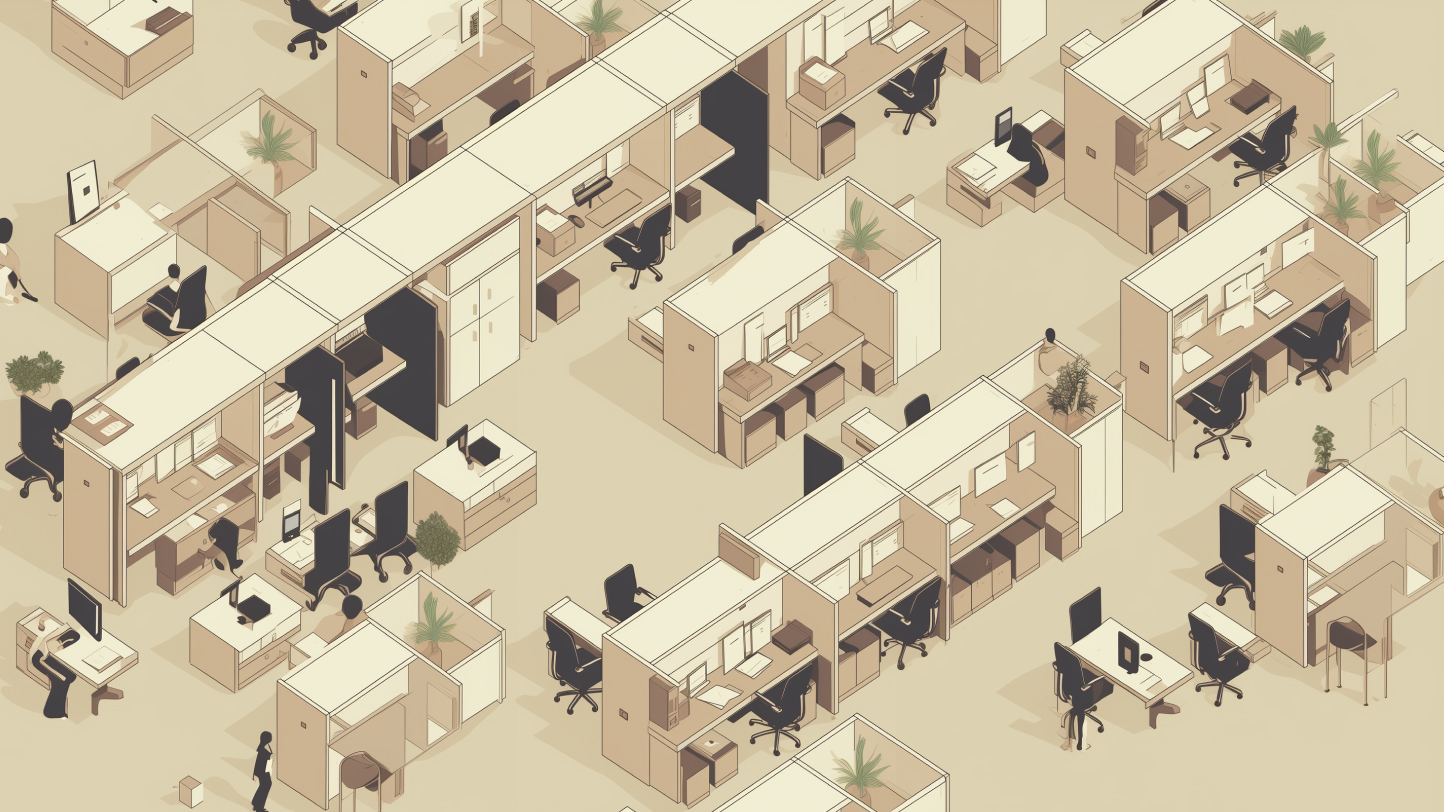
3/19/2024
The cubicle culprits: how in-office employees are leading the charge in corporate data exfiltration
In the wake of evolving work arrangements, the spotlight often falls on remote and hybrid employees as potential threats to data security. Yet, our latest research uncovers a surprising twist in the narrative. It’s the in-office employees, traditionally considered the safest bet, who are now leading the charge in corporate data exfiltration.
Thank you! Your submission has been received!
Oops! Something went wrong while submitting the form.

Live demo
See our product in action
The best way to understand the magic of Cyberhaven is to see a live product demo.
Request a demo